
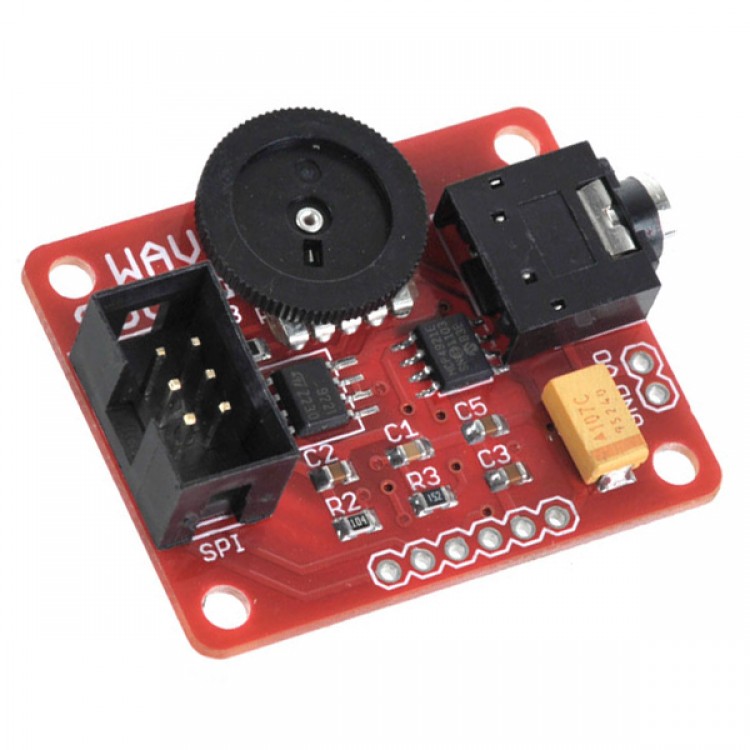
Most proposed techniques in the literature are applied during the IM startup transient, making it necessary to develop more efficient fault detection techniques able to carry out fault identification during the IM steady state. Broken rotor bars (BRBs), which are among the most regular and most complex to detect faults, have attracted the attention of many researchers, who are searching for reliable methods to recognize this condition with high certainty. Abstract: Induction motors (IM) are key components of any industrial process hence, it is important to carry out continuous monitoring to detect incipient faults in them in order to avoid interruptions on production lines. Broken Rotor Bar Detection in Induction Motors through Contrast Estimation. This method provides a new approach for weak fault feature detection in vibration and sound signals, and is of great significance for the maintenance of rolling bearing systems.Ĭitation: Ferrucho-Alvarez, E.R. A cascaded bistable stochastic resonance system is applied in the post-processing of the fusion signal to make the signal features more clear, and it is proved that the fault detection effect has an obvious improvement after the whole process. Results show that the two-step fusion provides a larger signal-to-noise ratio, and the amplitudes of characteristic frequencies are also higher. Then a second fusion between the vibration signal and sound signal is conducted after normalization and superposition, and the performance of two-step fusion is compared with the existing direct fusion. The sound signals are fused through weighting to the vibration signal to reduce the influence by measuring positions, and the phase difference is eliminated by a sliding window on the time axis. This paper puts forward a two-step vibration-sound signal fusion method, in which sound signal fusion and vibration-sound signal fusion are executed respectively. The vibration and sound signals get widely applications in fault diagnosis of rolling bearing systems, but the detection accuracy is unstable at different measuring positions. The results made it possible to obtain the failure diagnosis for 100% of the cases for both WMA and SAC-DM methods, but a shorter time window when using the last one. Experimental tests were carried out in a car to validate the techniques for the engine without failure, with failure in one piston, and with two failed pistons. In this work, two algorithms are compared, based on Wavelet Multiresolution Analysis (WMA) and another using an approach performing signal analysis based on Chaos using the density of maxima (SAC-DM) to identify misfare in a combustion engine of a working automotive vehicle. One of the most common failures in engines is called misfire, and it happens when the spark is weak or inexistent, compromising the whole set. Third-party devices and skilled labor are necessary to manage the data for failure characterization. Although modern vehicles are equipped with a failure detection system, it does not provide an online approach to the drivers. These failures cause inconveniences to the passengers and expose them to the dangers of the road. In the industrial Internet of Things, the conclusion can improve certain reference value for the future fault diagnosis of motor bearings.īesides the failures that cause accidents, there are the ones responsible for preventing the car’s motion capacity. At the same time, unlike previous studies, we found in our study that feature extraction does not improve the accuracy of diagnosis for the decision tree with ensemble method for AdaBoost and there is a precipitous decline. The accuracy of this method is as high as 99.9%. The results show that the optimal method is the Bayesian optimized decision tree with ensemble classifiers after feature extraction.
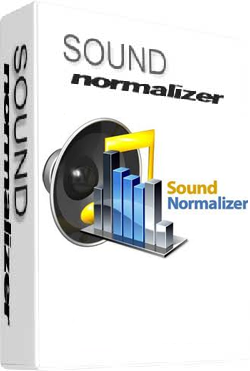
We derived four sets of experimental data. We use multiple feature extraction to establish the feature matrix and construct a decision tree model with the ensemble method for AdaBoost and a Bayesian optimized decision tree model with ensemble classifiers to conduct experiments on the accuracy, prediction speed, etc., of the model. In this article, the Bayesian optimized decision tree with ensemble classifiers after feature extraction of the original data is finally proposed which has good performance.
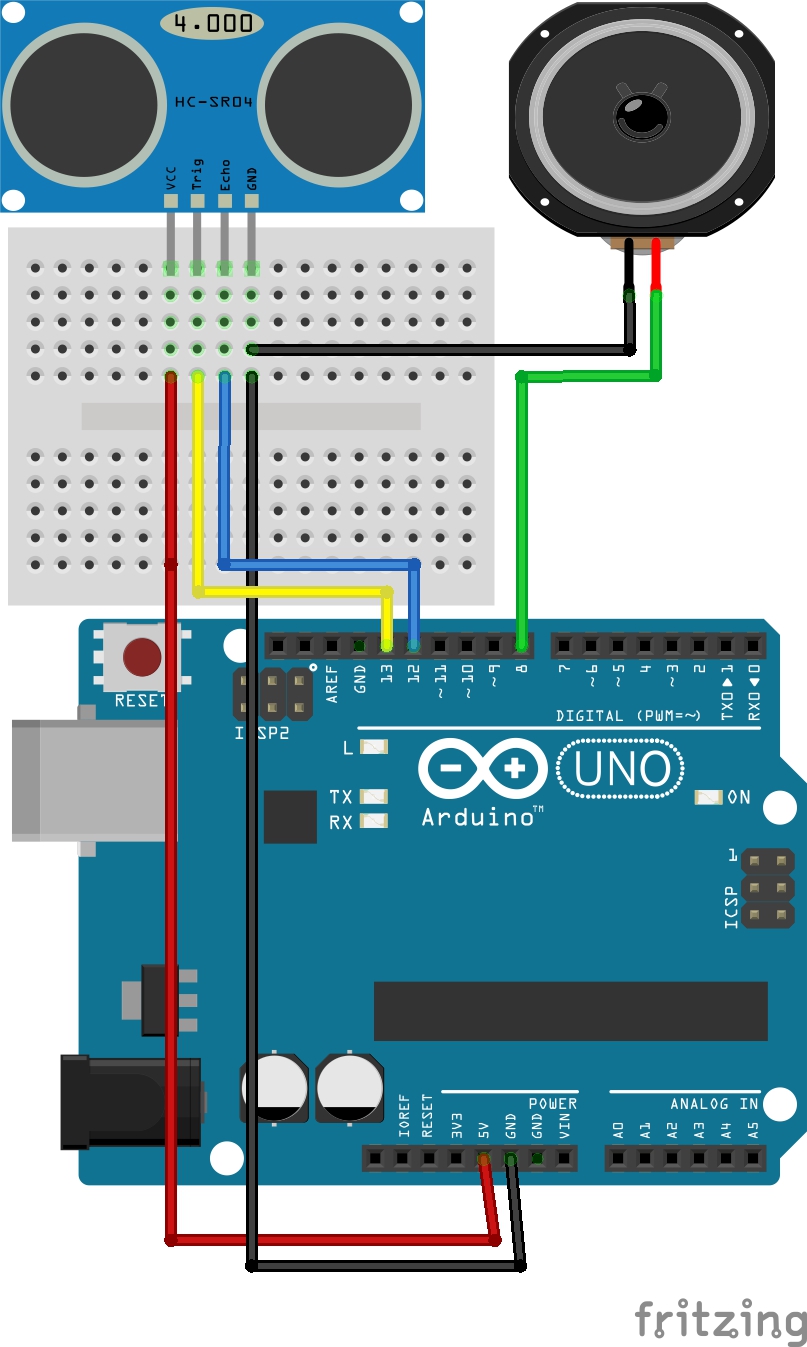
Most traditional methods for motor bearing fault diagnosis use a single learner and emphasize the role of feature extraction, which usually requires a large amount of sample support and computer runtime to obtain satisfactory performance. Nowadays, timely diagnosis of motor bearing faults is urgently needed. In order to adapt to the development of the industrial Internet of Things, the relationship between the internal components of electromechanical equipment is getting closer and closer, such as motor bearings.
